Prediction is very difficult, especially about the future*
- mariademertzis
- Feb 1, 2016
- 8 min read
The main issue at hand is our ability to predict and prepare for the future. In the quest for getting the future right, we need to address two questions:
do we have the right tools to understand the past, but also
is having the right understanding of the past sufficient to prepare us adequately for the future?
There is no doubt that knowledge and understanding of what has happened is important and useful. But we need also to acknowledge that the past will not always be a good predictor of the future. Typically, it is at these moments that our tools fail us, and they fail us quite dramatically. Policy response needs to both target the known unknowns and prepare for the unknown unknowns (radical uncertainty if you will).
1. How good are our tools?
Do we need models?
Yes we do. Models are tools that help us build an argument in ways that are internally consistent and structured. Above all, models enforce discipline to remain on one line of argument and importantly be very clear whether and how to deviate from it.
Economics and finance have in the past 20 to 30 years become increasingly mathematical and complicated. This is good because quantitative tools provide a consistent approach to argue a case. However, this has also pushed us to the comfort of believing that just because models produce numbers, these numbers are correct! The tendency has been for models to become bigger and deeper pushing us into a certainty that we now know was false. By losing tractability we compromised the most important contribution of models which is to guide in small steps.
Do we have the right models?
The questions that the organisers raised are suggestive of the fact that the models we used prior to the crisis were wrong. I will not disagree with that but I would like to express a rather more subtle view. Any given model is a thought experiment that cannot replicate the complexity of the economic system. If you believe that, then no model is really wrong; it is just more or less appropriate for the question at hand. So more than models being wrong, it was mostly the way that we used them that was wrong. We forgot their limitations and the real notion of model “errors”.
But I agree those models that we did use and relied upon failed us in a number of ways.
Where have our models gone wrong?
Models are linear when the world is typically not. New generations of models need therefore to allow for the possibility of non-linearities: for example, herding behaviour that may lead to bubbles in the upturn, in markets like housing which is of huge macroeconomic relevance. At the same time, they need to allow for the possibility of sudden stops in the downturn, generated by sustained deficits and excessive debt positions.
Agents have common or different beliefs but they do not interact. This makes aggregation of agents trivial. New models will need to account more and more for heterogeneous interacting agents, in which aggregate behaviour is more than just 'the sum of its parts'.
The irrelevance of banks in the macroeconomy. If one looks at the way that macroeconomics and finance evolved in the past 20 years, they could be excused for thinking that they are parts of two parallel universes, with no influence on each other. Banks did not affect the macro environment and macro policy (taxes, interest rates…) did not affect bank decisions. I may be exaggerating a little but only just a little. The profession is now attempting to change that and explicitly acknowledge that banks can affect the macroeconomy, and macro policy can affect the behaviour of banks.
Expectations are rational and therefore we cannot be persistently wrong. But this was more a matter of convenience than an intellectual necessity. The concept of bounded rationality is gathering momentum as we understand the systems to be complicated and uncertainty to prevail. But how does one tame the 'wilderness' of bounded rationality? When agents are different, is there a limit on how different they can be? A number of approaches have been used previously amid effort to address that and are gathering momentum.
The literature on learning has allowed for the 'postponement' of rational expectations. Agents are eventually rational (in that expectations converge to the 'right' outcome) but they are making mistakes in the short term, which they only correct every period as they slowly learn about shocks that occur.
Others have abandoned the idea of rational expectations altogether. The literature on "heuristics" , i.e. the application of simple rules or 'rules of thumb', is gathering momentum as a way of dealing with uncertainty. Simple rules, rules that are clear and transparent to relevant agents, can be effective as they do not add 'policy uncertainty' to the overall level of underlying uncertainty.
The importance of simplicity notwithstanding, there is on the other hand, also a tendency to acknowledge the complexity of economic phenomena. The literature on networks and experimental (behavioural) economics has attempted to understand the way agents interact and are therefore interconnected. Important in all this is treating the economy complex adaptive, nonlinear, evolutionary systems. This is an attempt to both acknowledge that on the one hand, non-linear relations govern economic phenomena but also that agents are faced with limited capacity to process, on the other. Simple rules, again, allow agents to learn in small steps the degree of underlying complexity, thus depart from the rational expectations' 'straight jacket' but not lose sight of direction.
Do failures identified imply the end of modelling?
Quite the contrary. We need more models not less: models that vary in breadth, depth, objectives and data congruency and are as varied as we can realistically afford. Small models for implementing thought experiments, big models for attempting to proxy the concept of general equilibrium, data congruent models to understand history, theoretical models to understand mechanisms and any combination of the above to address specific questions.
Model ambitions will define their nature. But there are important trade-offs to be reckoned with: as we gain in forecasting power we may lose in story telling; if we want more variables we may lose in sophistication of the relationships. And if we want both more variables and more sophisticated variables we may lose in tractability.
Importantly, we need to move away from the “certainty” of one model. In fact using one model is even more dangerous than using none, as one can get easily trapped in the false sense of security that “uniqueness” provides.
Equally important is to keep models tractable. This is the only way, in my view, in which models are useful. As soon as they become black boxes they lose their ability to tell an internally consistency story. I remind you: not a perfect story; not necessarily a story that corresponds to the truth that we observe. But a story that can help inform and understand mechanisms at work.
Last, let us not forget that models are crucial tools to establish best practice and to help keep policy makers accountable for their decisions. Losing them would risk making the policy decision process subject to political expediency. That said, models cannot and should not pass value judgments. Policy makers need to do that and that is why models only complement, never substitute judgement. There will always therefore be a need for having talent at the “driver seat”.
2. Immeasurable uncertainty
But in forming policy, that is only part of the story. This is the easy part that can help us prepare for the things we can measure and assess.
How do we prepare for things that we cannot measure, the unanticipated change?
Models cannot capture unanticipated change, almost by definition. It is the analysis around the models, typically what we call risk analysis that attempts to shed light into unanticipated change. And this analysis is a direct response to the fact that our models are imperfect.
Next to that, it is also true that the past is not always a good predictor of the future and that irrespective of how perfectly our models describe the past, they will not necessarily predict the future as accurately. But this implies that the probabilities based on which we typically rely to derive policies can be uninformative. And it is precisely at these moments, that our models fail us and fail us spectacularly. But if we cannot ex ante tell when these probabilities are informative and when they are not, can we base policy making on underlying probability distributions at all?
My view here that we need to de-emphasise the relevance of probabilities, how likely events are, and put more emphasis on preparing for a number of events, the impact of which we know to be big. Dutch engineers do not build damns based on likely rainfall; they distinctively build damns for very unlikely rainfall, but one that could have catastrophic impact. And since it is difficult to measure these probabilities in any other way than just frequencies, (i.e. historical occurrences), policy effort that aims to identify the impact of certain events instead, is put to better use.
To this end, there are two steps to policy decisions under radical uncertainty:
Identify the circumstances for which policies should be prepared for and by implication, what types of circumstances policies will not be prepared for!
Then understand that policies that will prepare us for these chosen events, are subject to a very important trade-off: that between performance and robustness. Imagine you are interested in building a car. If the fastest car is the only thing that you are interested in, then this car will only fair well in a set of very restricted conditions: namely good weather, good roads, no turns…etc….If you are interested in slightly more robust cars, that can do well in a wider set of circumstances, then you need to compromise in performance. Q: Then, of the different types of cars we could build which one should we choose? A: That car that will deliver the best performance for the widest set of relevant (weather, road…) conditions (given budget constraints). That performance will be good enough but it will not be the fastest car you could build.
Consider the design of financial architecture. We want banks to intermediate, take risks, invest, and contribute to growth. When economic circumstances are favourable, banks make profits and it is easy for them to perform their tasks. Moreover, it is possible to design regulation that will allow banks to perform their tasks optimally. But we also want banks to be able to operate in unfavourable circumstances that are hard to predict. This is why we put in place regulation that aims to increase bank resilience. When preparing for uncertainty our architectural choices reflect the trade-off between performance and robustness. If regulation (in the form of high capital ratios, low leverage ratios etc) aims for banks to withstand very unfavourable circumstances, banks will also be less capable to generate profits and perform their tasks under favourable circumstances. And the greater the range of circumstances that we want banks to be able to withstand, the less banks will be able to intermediate in normal time.
On the monetary side, I see the discussion on increasing the inflation target also in the context of managing uncertainty. It is true that if price stability is our objective, a higher inflation objective than the current one (typically around 2% in this part of the wold) will not deliver that. It will not cause rampant price increases but it will move us away from price stability. But what it will deliver at the same time is a better buffer from the very distortionary effects of disinfation, and ultimately deflation, and avoid the zero lower bound better. This in itself has value and ought to be considered. So such policy would deliver less good performance in good times but would also do satisfactorily (not optimally) in bad times.
3.Three steps to robust policy making under radical uncertainty
Going forward, and with the aim of using consistent frameworks while managing uncertainty, policy needs to proceed in three steps:
use models and judgment to form a best guess in terms of how the economy operates;
then decide which circumstances, beyond those anticipated, policy needs to be prepared for;
last, choose those policies that produce good enough outcomes for the widest possible set of circumstances
*Attributed to Niels Bohr a Danish physicist who received the Nobel Prize for Physics in 1922. This attribution is not indisputable though.
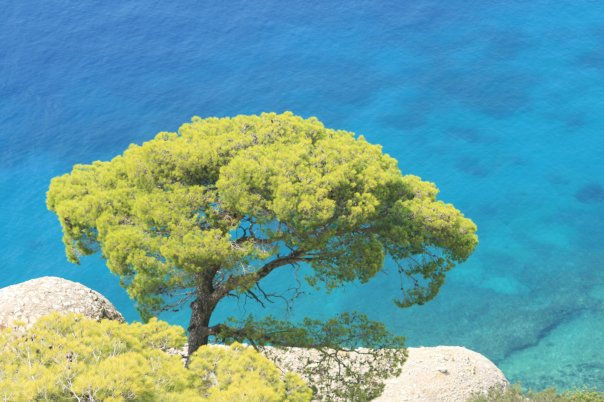
Comentarios